The NYT's Partisan Tale about COVID and the Unvaccinated is Rife with Sloppy Data Analysis
The Times' piece on "Red Covid" obscures the reality of the pandemic and manipulates data in favor of a self-congratulatory liberalism.
NOTE FROM GLENN GREENWALD: The corporate media has worked very hard to propagate the liberal-pleasing narrative that COVID has become a partisan disease due to vaccine hesitancy on the right, often ignoring the inconvenient truth that large percentages of politically diverse groups, principally African-Americans and Latinos, remain resistant to vaccination. Recent reporting from The New York Times serves to further this distorted narrative, dubbing the positive correlation between support for Donald Trump and COVID death rates “Red COVID," and brandishing it as evidence of a partisan pandemic. But the Times’ report misleads readers through statistical manipulation and data games, as illustrated by this meticulous analysis, presented in an Outside Voices contribution by Jeremy Beckham:
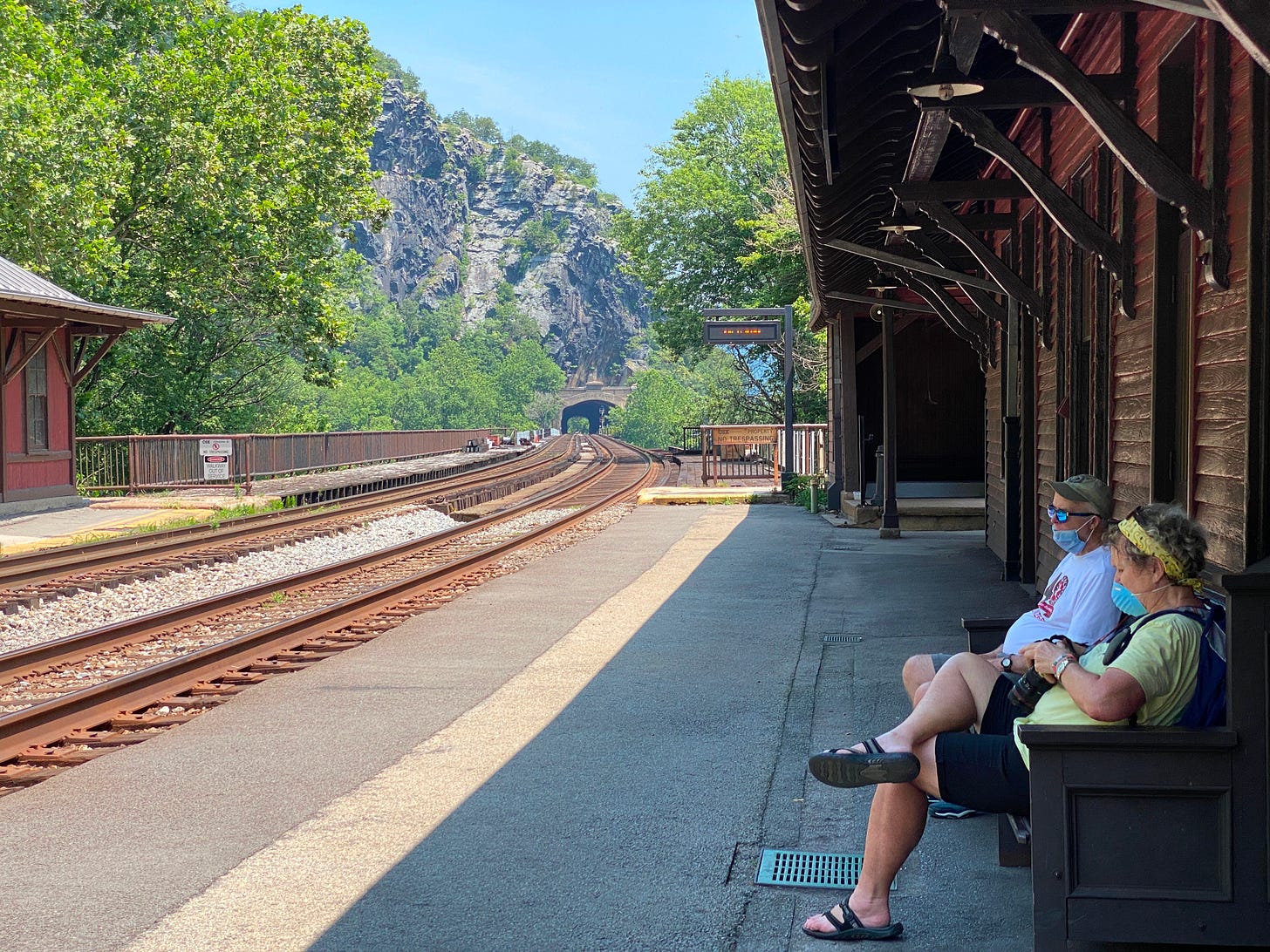
By Jeremy Beckham
A widely shared article recently appeared in The New York Times’ “The Morning” newsletter titled “Red Covid,” authored by David Leonhardt. This article, presented as news reporting and not an opinion piece, argues that deaths from COVID-19 are “showing a partisan pattern,” with the worst impacts of the disease “increasingly concentrated in red America.” Given that this narrative perfectly flatters a liberal sense of superiority, it has predictably gained substantial traction on MSNBC and on Twitter.
One particular claim in the Times' article caught my attention: that there is a clear and strong association on a county level between COVID deaths and support for Donald Trump in the 2020 election. Specifically, the article alleged that those counties which voted overwhelmingly for Donald Trump had more than a four-fold greater mortality rate than those counties which decisively voted against Trump. If true, that would indeed be a striking observation.
But, as is often the case with epidemiological observations, the question is more complicated than two variables. There are three analytic errors that can lead someone to make false conclusions from what appears to be a meaningful association between two variables: bias, confounding variables, and random statistical error. In this case, the Times’ analysis failed to discuss significant confounding variables.
Age is a common confounder in public health research, and COVID-19 is no exception. The mortality burden of COVID-19 is not randomly distributed across age groups. Indeed, age appears to be the “strongest predictor of mortality” from COVID-19, with one’s risk of death increasing exponentially with age. According to CDC figures, the oldest populations experience a rate of death 570 times higher than that of the youngest populations. This is precisely why older populations were vaccinated first; we knew that prioritizing this population would have the most dramatic effect in curtailing hospitalizations and deaths. Yet the crude county-level analysis reported in The New York Times failed to adjust or account for age at all.
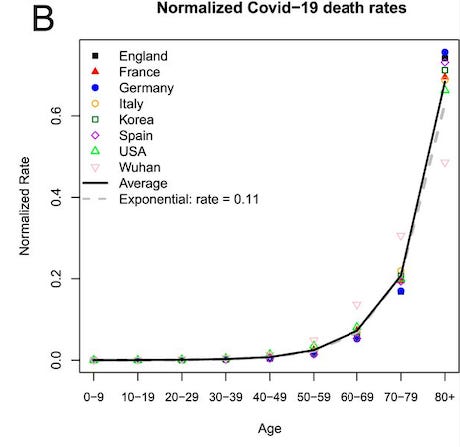
Why is it especially important that we adjust for age when comparing COVID-19 mortality rates in “red” counties with “blue” counties? Because age is not randomly distributed geographically, nor is it randomly distributed on a partisan basis. Republican voters tend to be older than Democratic voters. And rural counties, where Trump won by the largest margins, have older populations than suburban and urban counties. So this means that age is clearly a third, unaccounted for factor that is associated with both the independent variable (a county’s political affiliation) and the dependent variable (COVID-19 fatality rate) in question. This makes it a significant confounder that could easily exaggerate or distort the measured effect and lead one to spurious conclusions.
To be clear, age affects a wide range of health outcomes, and the presence of age as a confounder doesn’t necessarily preclude a subject from methodologically sound inquiry. But you do need to account for it typically by using a statistical process like age-adjusting. For instance, one study looked at seven different nations with widely different crude COVID-19 case fatality rates, ranging from 0.82% to 14.2%. However, once the study’s authors performed age-adjusting, they found that the difference in fatality rates between these countries almost evaporated. If this research technique is not feasible on the county level, perhaps because the available data is incomplete, then it’s important to explicitly state that a known confounder is a limiting factor in extrapolating the significance of your research, so that the reader knows to take the findings with a grain of salt.
Another way researchers try to tease apart a confounder from the variable being investigated is looking only at data where the distorting effect of the confounder is not present. We could do something similar for this research question. Take my home state of Utah, for example. Utah is a very red state. Trump won Utah by more than 20 points in 2020. But Utah also has the youngest population in the country, with a median age of approximately 31 years old. If partisan affiliation were a significant factor that explains deaths from COVID, we would expect Utah to have a greater COVID death rate than the national average, and the younger population helps us minimize the effect of this confounder. But instead, what we find is that Utah ranks 45th in the nation for COVID deaths, with 91 deaths per 100,000 population, far below the national average of 210 deaths per 100,000. This suggests (without proving) that age, not partisan affiliation or ideology, is paramount.
And all of this only accounts for one potential confounder (age). There are other potential confounders that should be addressed. For instance, the disparity in health outcomes between rural and urban populations likely means that people in counties that voted heavily for Trump have other comorbidities that place them at greater risk of death from COVID-19. And people who live in rural areas also experience significant disparities in health care access, with higher rates of uninsured, diminishing available health care facilities, and longer travel times to the nearest hospital. In the past decade, 138 rural inpatient hospitals have closed. This unjust inequity that persists in rural America has previously been a matter of persistent concern for writers at The New York Times, even in the context of reporting on COVID-19 when the pandemic was in its early stages.
To be clear: there is no question that COVID-19 vaccines are a safe, effective, and important tool in protecting people from severe disease and death. The vaccination rate for rural counties is 41.4%, while the rate in urban areas is 53.3%. This difference also surely has an impact on the different rates of death from COVID-19. But this is only one part of the equation, and The New York Times’ recent viral article contained no such nuanced or informative discussion about this complex web of interrelated factors influencing disease burden and health outcomes. If you search the article for any mention of ‘age,’ or ‘rural’ you get no results, because these factors didn’t appear in their analysis at all. In any discussion about factors influencing COVID-19 mortality rates, failing to mention the role of these important demographic influences is journalistic malpractice that grossly distorts reality.
So if it failed to account for any of these factors, how did The New York Times ultimately account for the higher death rate in Trump/rural counties? It does so entirely by invoking the ideological makeup of their knuckle-dragging residents and their apparent self-destructive desire to “own the left.” Under the subheading “Why is this happening?”, The New York Times asserts the following:
What distinguishes the U.S. is a conservative party — the Republican Party — that has grown hostile to science and empirical evidence in recent decades. A conservative media complex, including Fox News, Sinclair Broadcast Group and various online outlets, echoes and amplifies this hostility. Trump took the conspiratorial thinking to a new level, but he did not create it. “With very little resistance from party leaders,” my colleague Lisa Lerer wrote this summer, many Republicans “have elevated falsehoods and doubts about vaccinations from the fringes of American life to the center of our political conversation.”
Part of the problem is that The New York Times relied on incredibly shaky source material. Much of the article is based on writings of an individual named Charles Gaba, who appears to be a web designer and “internet consultant.” Gaba runs a Patreon page where he disseminates his writings to subscribers, which are not submitted to reputable peer-reviewed publications. But this lack of rigorous scientific review was no barrier for The New York Times relying on his "findings," even as the Paper of Record in the same breath scolds those who it says remain “hostile to science and empirical evidence.”
The irony is thick indeed, and the reason why should be obvious: even if the methodology is unsound, the findings fit a preferred narrative that the overwhelmingly liberal readers of The New York Times want to hear. If Gaba’s blog had made the opposite claim – if it had focused on counties like those in Utah, for instance – I think it’s safe to say it would have never seen publication in The New York Times. For this paper, it appears that feeding its readers' desire to feel intellectually and morally superior to “Red America” is of utmost importance, even if it comes at the expense of accurately reporting on the complex reality of the COVID-19 pandemic.
We may not be better informed, but at least we know who to hate.
Jeremy Beckham, MPH, MPA, is a Utah resident who writes on matters related to science, politics, and animal rights.
To support the independent journalism we are doing here, please obtain a gift subscription for others and/or share the article:
"To be clear: there is no question that COVID-19 vaccines are safe, effective, and an important tool in protecting people from severe disease and death."
The safety of COVID vaccines can indeed be questioned. The very paper that was supposed to demonstrate Pfizer vaccine safety indicated 101 "severe" adverse events caused by the vaccine compared to 8 cases of "severe COVID-19" prevented (see Tables S3 and S5).
https://www.nejm.org/doi/full/10.1056/NEJMoa2034577
Beckham's analysis is on point, but his article of faith statement that "there is no question that COVID-19 vaccines are safe, effective, and an important tool in protecting people from severe disease and death" undercuts his analysis. We do not have enough experience or data to affirm those claims yet, notwithstanding the political rush to approve. Little to no research is being done long term on side effect;, there is concern that issues are underreported on VAERS; there is still debate and experiment on how far apart the initial doses should be, and while it is clear that the efficacy of the mRNA vaccines wane relatively quickly, there is no data on the impact of repeated shots, or of the interaction between the vaccine and the antibody status of people who have had the disease, yet many employers are demanding mandates for vaccination, even among those with natural immunity from experience of the disease. Similar statements in many reports seem to me an attempt to maintain credibility with the establishment that is being challenged. it won't work, and we've had our thalidomide and Zantac and how many more experiences in the past were it took longer to see the problems. The political manipulation of COVID to enhance government and corporate power, and to advance an idea of safety, as defined by the corporate government axis as paramount over protection of individual rights, minority opinions, and autonomy over our own bodies is the core of this issue, not the efficacy of a vaccine.